C-Suite vs. Mid-Level: The AI Optimism Gap in GRC
As the mid-level employees work to implement their c-suite’s AI strategies, the two groups need to collaborate so that they can optimize the benefits while mitigating the risks.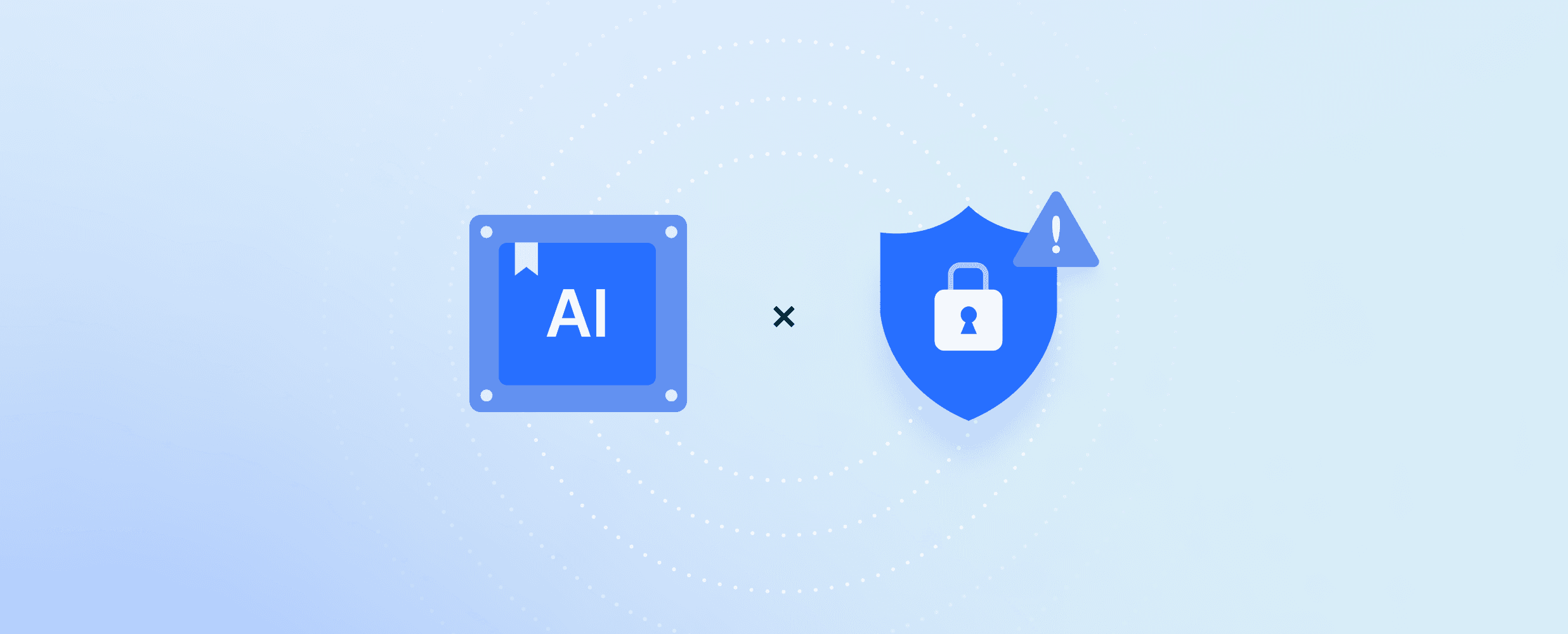
Many forms of public transportation warn you to “mind the gap.” While these small spaces between where a subway platform ends and the doorway opens can seem inconsequential, a small stumble can cause you to hurt yourself or knock other people over.
In business, the rise of artificial intelligence (AI) has created a new seemingly inconsequential gap that can be potentially painful to everyone. AI is a mixed bag for most companies. The senior leadership team views it as a way to streamline processes and save money. However, the security and compliance teams who have to work with these new technologies may struggle to manage the impact they have. According to the State of GRC 2025 report, 42% of c-level and senior executives are hopeful for AI’s impact, 30% of mid-level employees are hopeful for AI’s impact
As the mid-level employees work to implement their c-suite’s AI strategies, the two groups need to collaborate so that they can optimize the benefits while mitigating the risks.
Mind the (Optimism) Gap
Where senior leadership envisions AI as a helpful little droid like Star Wars’ R2-D2, security teams see a technology similar to The Terminator, something that could become dangerous if left uncontrolled.
Despite the push to incorporate AI into business operations, the State of GRC 2025 report found that only 10% of organizations are completely prepared to manage increased employee AI use. The two groups of business leaders view AI through different lenses, but understanding each side’s viewpoint can help them come to a compromise.
Efficiency vs. Security
Most companies digitally transformed their business models to improve operations and create efficiencies. For example, Software-as-a-Service (SaaS) models allowed people to work from anywhere and collaborate more effectively. Simultaneously, they expanded an organization’s attack surface with each new login required.
AI creates the same tension within the organization’s leaders. While 100% of respondents in the State of GRC 2025 report believe employees will increase their use of AI in the next 12 months, only 10% have a fully prepared GRC program for managing it.
Analytics vs. Transparency
Most AI models are functionally complex math that makes statistical predictions by ingesting large volumes of data. Often, organizations simply connect their technologies to the platform and allow the platform to run the analytics models. Senior leadership teams recognize the value of these models, with 40% predicting that they will enhance decision-making and predictive insights.
From the security and GRC perspective, this creates friction as many models are proprietary, giving customers outputs with little insight into the data they ingest. Regardless of the artificial intelligence or machine learning (ML) model’s purpose, many companies lose control over the sensitive data that the technologies use. Further, many products simply automate tasks rather than allowing companies to disable them, even when the AI/ML is a small part of the product’s business value.
Innovation vs. Governance
Being an innovative company often means using cutting edge technology to provide the best customer experience possible. Whether using AI to suggest items that match previous purchases or building AI into an app, senior leadership is always thinking about ways the company can gain a competitive advantage.
For the GRC and security teams, innovation still requires documentation. When asked about AI’s impact on the organization’s approach to GRC, 44% of respondents believe that it will cause a complete overhaul or massive impact. While the use of AI for the GRC function may streamline processes, it will require additional compliance as evidenced by recent publication of the NIST AI Risk Management Framework (NIST AI RMF) and Artificial Intelligence Risk Management Framework: Generative Artificial Intelligence Profile.
Understanding the Challenges of Managing AI
For the c-suite, AI is a technology that saves money across typical business operations. However, once you dig into the technology impact more, you can better understand why some security and GRC teams would be skeptical.
New Attack Vectors
As people use AI to complete repetitive tasks faster, they can create new security risks like:
Accidentally feeding sensitive information into models.
Connecting models with insecure application programming interfaces (APIs).
Using work credentials to log into public AI platforms.
Whether building an internal AI model or using someone else’s, security teams need to build these risks into their penetration testing. Beyond the typical concern around technical vulnerabilities, they have to scale up their ability to write and test prompt injection attacks.
Changing Risk Profile
AI implementations change the organization’s risk profile. Whether used in customer-facing applications or internally built GRC applications, these models manage sensitive data that increase risk. For every change in risk, these teams need to document their risk mitigation activities.
For example, DevOps teams need to document data flows into and across the AI model, including what data it ingests and how the tool handles sensitive information. By increasing the CI/CD pipeline’s complexity, maintaining up-to-date documentation to comply with secure software development lifecycle (SDLC) processes becomes even more challenging and time consuming.
New Third-Party Vendor Risks
Without insight into the data that the models use and how they manage it, security and GRC teams have new third-party risk blindspots. Using vendors with AI tools changes the questions that organizations need to ask and create new supply chain risks. For example, they need to ask vendors new questions around how they secure their models to verify the quality of outputs. They also need to know how the vendor tested for vulnerabilities, including prompt injection.
Inability to Extend Security Controls
AI models run on data, meaning that the security and GRC teams need to engage in due diligence to ensure that data pipelines protect sensitive information. Any data that the AI ingests is now potentially part of training the model. While a proprietary AI/ML solution may have security controls that align to your risk profile, public models may not. As employees use these tools, the security and GRC teams need to have guardrails that mitigate risks. Increasingly, they need to ensure that workforce members engage in AI awareness training, the same as they do cybersecurity awareness training.
Practical Steps for Bridging the Gap
AI is here to stay, and internal stakeholders need to come to a workable consensus of opinion about how to deal with it.
Identify Use Cases
AI implementations should be purposeful so that the security and GRC functions can implement the appropriate controls. When seeking to deploy AI, you should consider the use cases that make the most sense for your business objectives and risk profile. Some examples of use cases may include:
Automating risk analyses for real-time updates and insights.
Scheduling follow-up tasks and building workflows.
Summarizing information like vendor security questionnaires or security testing results.
Manage Sensitive Data
Depending on your risk appetite, you may want to allow an AI to analyze sensitive information. Simultaneously, you want to minimize the data that these tools ingest and understand the potential risks.
In this case, thinking like a GRC team member is helpful because you can consider different data minimization questions, like those from the General Data Protection Regulation (GDPR):
Is the amount of data adequate to fulfill your objective?
Is the data being used relevant to achieve the desired outcome?
Have you limited the amount of data ingested to only what is necessary?
Review for Customization
While AI can offer benefits, you want to have control over your data. Your risk appetite is unique to you, so you should look for AI tools with flexible configurations. For example, every AI tool should allow you to disable the AI features without compromising their value. AI should be an added bonus not a requirement, especially when it ingests and analyzes sensitive information.
Ensure Vendor Transparency
Vendors should be concerned about your data and be transparent about how they use it. When considering an AI tool, you should ensure that it appropriately protects data by:
Anonymizing datasets to preserve privacy, like masking sensitive data.
Following strict access control and encryption protocols to comply with data protection regulations.
Communicating the company’s privacy practices and data management protocols.
Enforcing strict data separate across all customers.
Designing AI with fairness, inclusivity, safety, reliability, and privacy in mind.
Regularly reviewing models and using automated tools to ensure data quality.
How Drata Enables Responsible, Purposeful AI Use for GRC
Drata’s GRC platform enables you to leverage AI responsibly by providing a platform that you can customize to your business operations needs and risk tolerance. Our platform provides:
AI features that include vendor questionnaire summaries, security testing summaries, and automation for responding to customer vendor questionnaires.
Custom risk scoring so you can define and configure your risk scores and thresholds to your business needs.
Automated treatment plans based on your unique risks’ impact and likelihood.
Custom frameworks so you can easily and quickly bring in requirements related to your unique business needs.