Trust and Privacy by Design: Drata's AI Philosophy
In the domain of compliance automation, safeguarding client data is not just a priority; it is the essence of trust and service integrity.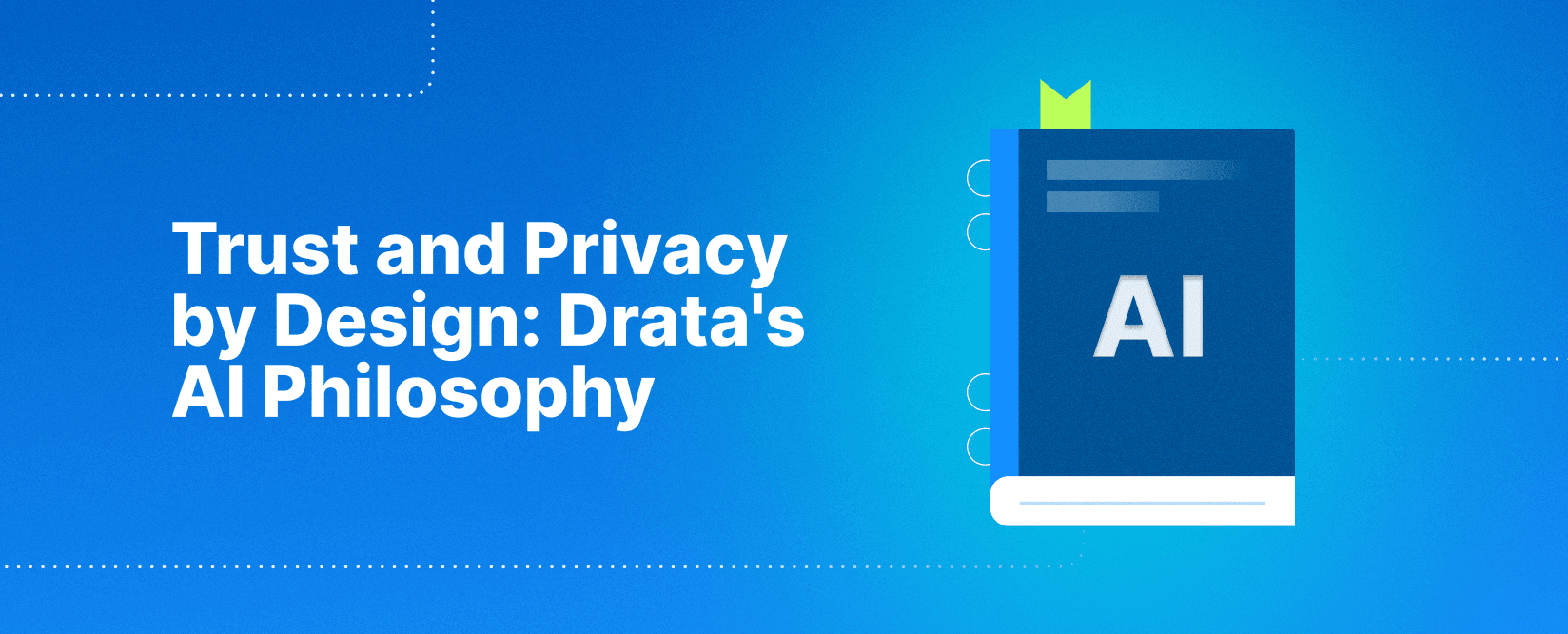
In the domain of compliance automation, safeguarding client data is not just a priority; it is the essence of trust and service integrity. In this space, the implementation of AI transcends ethical usage; it demands an ironclad guarantee that risks are meticulously managed and that customer data remains confidential and secure.
Moreover, we understand that regulations and guidance are catching up with the available technology, but the temporary wild west scenario does not offer an excuse for people and organizations to misuse it in a way that negatively impacts society.
Prioritizing the Ethical Use of Data in AI
While AI offers transformative potential for decision-making and efficiency, our unwavering commitment to risk management and data security forms the bedrock of our customer relationships. This focus on security ensures that AI systems are not only advanced and ethical but also resilient against breaches—upholding our promise to protect our customer’s interests above all else.
To that end, below are our core principles of trust and safety in AI development:
Drata's Core AI Principles For Product Development
These core tenets of fairness, safety, reliability, and privacy guide both our organizational values and AI development practices. By embedding such responsible AI principles across our systems and processes, we uphold robust governance ensuring our solutions empower users ethically.
Privacy by Design
Protecting sensitive user data is the foundation of trust in AI.
At Drata, here’s how we’re protecting customer data:
We anonymize datasets to preserve privacy through strategies like data masking.
We follow strict access control and encryption protocols aligned to global data regulations.
We clearly communicate our privacy practices and data management protocols to users.
Our privacy by design principles reinforce Drata's commitment to earning user trust through responsible data stewardship.
In addition, we leverage synthetic data generation to simulate a wide range of compliance scenarios. By training models on this realistic generated data, we strengthen reliability by accounting for diverse situations—all while protecting the privacy of actual user data.
Fairness and Inclusivity
We believe AI systems should be fair, inclusive, and accessible to all.
To achieve this, we:
Carefully curate and preprocess datasets to remove biases.
Continuously monitor our models for unfair outcomes or exclusions and refine them accordingly.
Design intuitive interfaces allowing users of all backgrounds to leverage AI meaningfully.
Our tools provide clear explanations around AI reasoning and guidance for non-experts to support understanding and accessibility.
In addition, we consciously build solutions suited for diverse business environments—from early-stage startups to mature enterprises. By accounting for varying levels of resources, data maturity, and compliance expertise, we advance inclusivity and accessibility by ensuring the benefits of AI are not exclusively based on company size and scope.
This emphasis on equitable access is central to our mission of making sophisticated AI solutions inclusive for organizations of all sizes and sectors. We are committed to maximizing AI’s democratization potential through both our technological design and educational outreach focused on marginalized communities.
Safety and Reliability
As AI permeates decision-critical functions, ensuring these systems are safe, secure, and reliable is imperative. We:
Subject all AI models to rigorous testing, including evaluation on synthetic datasets reflecting diverse scenarios.
Enable human oversight mechanisms providing users transparency into AI workings.
Have well-defined model monitoring procedures to evaluate ongoing performance.
By prioritizing safety and reliability, we provide assurances to users dependent on AI that these systems will function as intended despite real-world complexities.
Our AI Perspective and Related Definitions
Underpinning Drata's mission to Build Trust Across the Cloud is a deep understanding of the evolving role of compliance professionals. In this dynamic environment, Artificial Intelligence (AI) is a vital tool, not just for automation, but as a strategic ally.
By taking on routine compliance tasks, AI streamlines routine tasks for professionals, allowing them to focus more of their expertise and time on strategic, long-term decision-making; focus on building a culture of security and compliance.
Before we outline the potential impact of AI on compliance automation, let’s highlight why now is a pivotal moment for its adoption and agree on terminology.
Artificial intelligence (AI), machine learning (ML), large language models (LLM), and diffusion models have been in development and the focus of academic research for many years. Recent advancements, particularly in Amazon Bedrock's array of models such as AI21 Labs' Jurassic, Anthropic's Claude, Cohere's Command and Embed, Meta's Llama 2, Stability AI's Stable Diffusion, and Amazon's Titan models, showcase the remarkable progress in AI capabilities.
These developments, combined with improved training data availability, enhanced computing power, and the expansion of generative AI's scope, have led to an explosive growth in AI applications. This burgeoning landscape emphasizes the need for organizations to develop strategic plans for engaging and utilizing AI within their operations.
A clear understanding of the various aspects of AI, including machine learning and generative AI, is also essential for comprehending their impact on industries such as compliance automation. Additionally, this understanding will be pivotal as we later discuss the specific security considerations we have implemented for each aspect of AI in our systems.
Let's break down these terms to understand the landscape and lay the groundwork for our discussion on security.
Artificial Intelligence
Artificial intelligence (AI) is a broad term that encompasses all fields of computer science that enable machines to accomplish tasks that would normally require human intelligence. Machine learning and generative AI are two subcategories of AI.
Machine Learning
Machine learning (ML) is a subset of AI that focuses on creating algorithms that can learn from data. Machine learning algorithms are trained on a set of data, and then they can use that data to make predictions or decisions about new data.
Large Language Model
A large language model (LLM) is a type of AI program that uses machine learning to perform natural language processing (NLP) tasks. LLMs are trained on large data sets to understand, summarize, generate, and predict new content.
Generative AI
Generative AI is a type of machine learning that focuses on creating new data. Often, this relies on the use of large language models to perform the tasks needed to create the new data.
Responsible AI in Action
At Drata, responsible AI extends beyond lofty aspirations into tangible, rigorous governance in practice.
In this section, we’ll lay out those governance policies and procedures in action through concrete examples across our AI systems and development lifecycle. From tenant-specific machine learning models to strict generative AI content controls to robust data anonymization mechanisms.
Our aim is not only to build state-of-the-art AI solutions, but to do so in a way that proactively earns customer trust through accountability. By detailing these responsible AI implementations in action, we offer both ethical assurances today and a blueprint for the future stewards of this transformative technology.
Dedicated Machine Learning Models for Data-Specific Insights
In our steadfast commitment to security and privacy, we at Drata prioritize the sanctity of your data.
Recognizing the unique nature of each customer's environment, our machine learning models can be designed to be tenant-specific. This means that when we train our AI systems, we use only your data, ensuring that the insights and intelligence gleaned are exclusive to your organization.
This approach not only strengthens data privacy but also fortifies the security of your information. By segregating machine learning models and datasets, we eliminate the risk of cross-contamination between different customers' data. Each customer's model is a self-contained unit, benefitting solely from their data, thereby maintaining the integrity and confidentiality that you expect and trust.
While we focus on protecting and utilizing your data responsibly, we also harness the power of AI to enhance the compliance automation services we provide. Our tenant-specific models are more than just a technical feature; they are a promise of personalized security and privacy in the cloud.
Enhanced Machine Learning Through Collaborative Data Enrichment
In our quest to democratize access to advanced machine learning capabilities, we've adopted an innovative shared model approach. This strategy is particularly beneficial for clients who may not have the extensive datasets typically required to train effective AI models independently.
Here's how it works:
We consolidate anonymized datasets from various clients into a central repository. This data pooling strategy is crucial for those with less data, as it allows them to benefit from the 'collective intelligence' of a broader dataset. Rigorous anonymization processes are employed to ensure the utmost privacy and compliance with relevant data protection regulations, effectively removing any personal or identifying details from the data.
The anonymized data then serves as the foundation for a powerful, pre-trained model that all clients can utilize for their unique inference needs. This shared AI model is capable of delivering sophisticated insights and predictions, contributing significantly to each client's product outputs.
Such an approach not only enhances model accuracy but also fosters an environment where all clients, regardless of their size or data maturity, can leverage AI to its full potential. And rest assured, the integrity of each client's sensitive information remains intact, protected by state-of-the-art anonymization techniques.
This approach allows us to eliminate the known cold start for new customers that are looking to utilize the shared knowledge of models that have been used and trained with other customers.
For example, imagine uploading a policy and getting a recommendation to which controls it should be mapped automatically. An early-stage startup doesn’t necessarily have a compliance team, so they greatly benefit from recommendations generated by our recommendations model trained on past manual mapping done by other customers.
Responsible Governance of Generative AI
As generative AI powers innovations from content creation to personalization, establishing proper governance is crucial. At Drata, we implement rigorous controls around our generative AI systems:
Strict Data Separation
In our approach, we leverage a retrieval-augmented generation (RAG) stack, ensuring that each vector database is exclusively allocated for a specific customer. When utilizing our language learning models (LLM) to answer queries based on these vector databases, we employ a segregated version uniquely dedicated to each customer.
This architecture not only upholds strict data privacy by keeping customer data distinct and secure but also guarantees the generation of high-quality AI outputs, tailored to individual customer needs.
Content Moderation Guardrails
We configure multiple guardrails to filter out harmful generative content, including:
Denied topic lists flagging undesirable categories.
Thresholds blocking toxic language, insults, and hate speech.
Tools scanning for violence, sexual material, and more.
Regular Audits
Both human reviewers and automated tools continuously audit generative content for policy violations we may have missed. This allows us to quickly refine content filters to align with our ethical standards.
Geographic Data Restrictions
For customers requiring data localization, we provision region-specific generative AI services. This includes configuring certain models to ensure data remains stored within GDPR-compliant regions.
Through these best practices for data separation, content control, auditing, and geographic restrictions, we safeguard responsible governance over generative AI capabilities.
Our commitment to ethical AI applies robust security measures not just to raw data, but also the synthetic content produced using that data. Maintaining trust and integrity across the generative pipeline is essential as AI becomes further embedded in critical workflows.
Technology Builds Best When Built Together
At Drata, responsible and ethical AI is not just a lofty idea but a practical commitment woven through all aspects of our platforms. From tenant-specific machine learning to generative content guardrails to stringent data privacy schemes, we implement comprehensive governance reflecting industry best practices.
These rigorous controls deliver tangible dividends for our clients. Our focus on fairness and inclusivity promotes accessibility of sophisticated AI regardless of resources. Our obsession with reliable and secure models provides the trust needed to embed AI in critical workflows. And our privacy-preserving data strategies reinforce customer confidence and peace of mind.
Beyond individual benefits, our responsible AI approach aims to raise the bar for the entire ecosystem. As an emerging leader in compliance automation, the precedents we set can positively influence AI governance across sectors. And our commitment to transparency provides a blueprint for the responsible innovation imperative of our age.
At its essence, Drata's pursuit of ethical AI stems from a simple belief: that technology builds best when built together. We consider it both a privilege and duty to steward AI's development in a way that distributes gains fairly and earns trust broadly. Because only by championing responsible progress for all can we unlock the full potential of what this technology promises. We're excited to realize that promise in partnership with our customers and community.